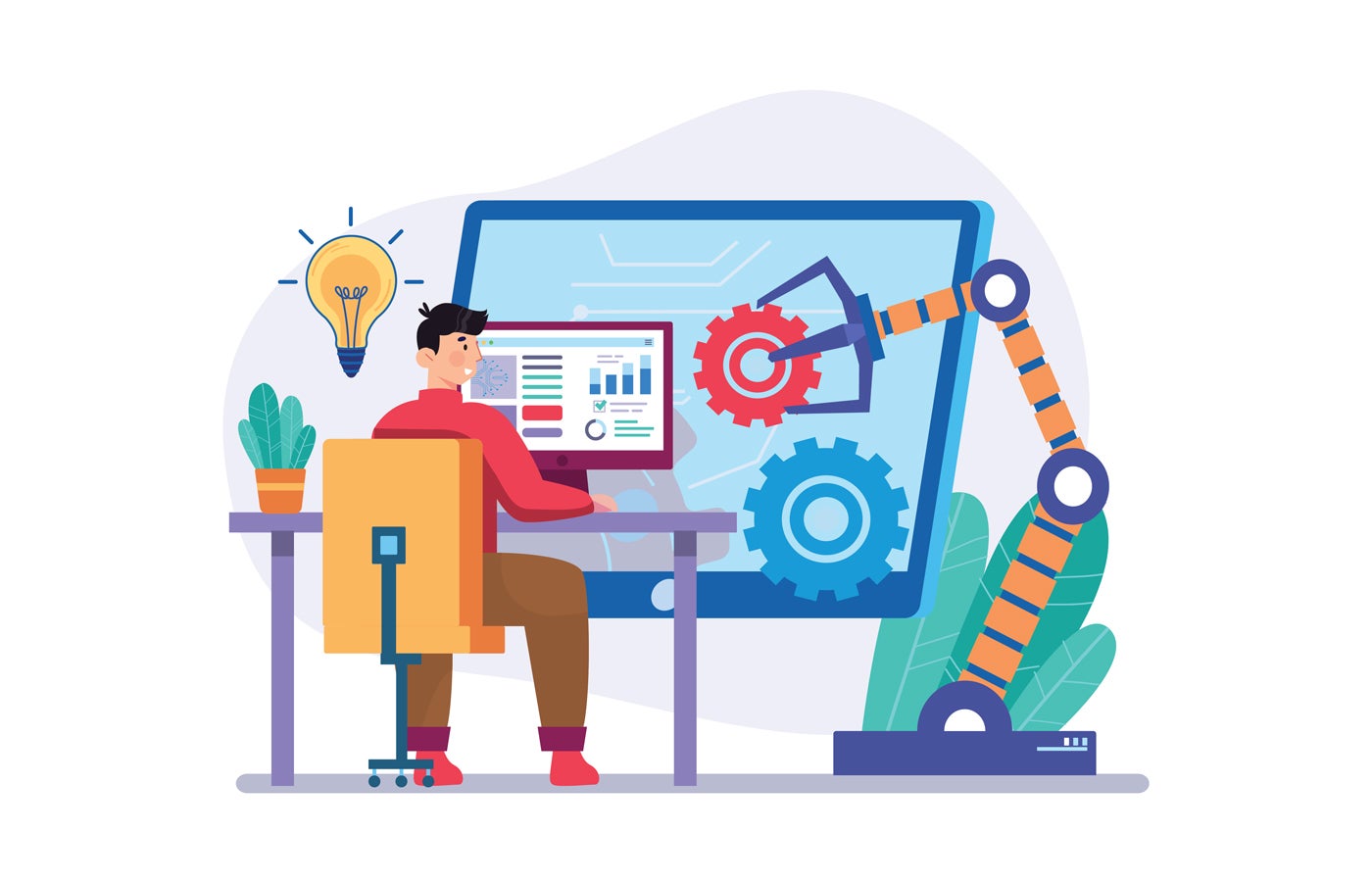
The trajectory of enterprise technology has often been marked by fragmentation. In the past, the rapid expansion of data platforms led to a fragmented ecosystem as vendors rushed to support various data types and tools. For instance, organizations often manage structured data with relational databases like MySQL or Oracle, semi-structured data with NoSQL databases such as MongoDB, and unstructured data with data lakes implemented with Hadoop or Amazon S3. Big data processing frameworks like Apache Spark were then layered on top to manage large-scale data analytics. The result? Complex, costly systems that were difficult to maintain and failed to deliver seamless insights.
Today, a similar scenario is unfolding with AI. The explosion of predictive, generative, and agentic tools has created a fragmented landscape where businesses struggle to integrate multiple solutions effectively. Managing these isolated AI capabilities separately increases complexity, reduces efficiency, and limits the full potential of automation. A unified AI stack solves this problem by consolidating AI-powered automation into a single, cohesive ecosystem.
In customer service, for example, a company may want to combine predictive AI to anticipate customer issues, generative AI to create personalized responses, and agentic AI to autonomously handle complex interactions. This integration allows for a seamless and intelligent customer support system that reduces human workload, enhances customer satisfaction, and improves operational efficiency — delivering on the true promise of AI. However, with fragmented AI tools, this type of real-world scenario becomes very complex and costly to deliver, requiring licensing, training and deploying multiple different AI tools and solutions. This complexity gets in the way of business innovation and impedes your progress toward strategic outcomes.
To reduce complexity and unlock AI’s full potential, organizations should take a strategic approach to integrating AI across their operations. This requires not only consolidating AI tools but also establishing governance frameworks to ensure long-term success.
How to manage AI fragmentation: Consolidate AI tools and frameworks
For fear of missing out, some organizations jumped the gun and adopted AI as soon as GenAI hit the mainstream in 2022 following the release of OpenAI’s ChatGPT. These early innovators are now dealing with a patchwork of disconnected solutions that have led to redundancies, inefficiencies, and maintenance challenges. While each AI tool may provide value on its own, fragmented systems create unnecessary complexity that slows down innovation. For those companies looking to streamline their AI strategy — or those considering new AI investments — the path to a resolute AI stack is rather straightforward; assess the current AI ecosystem and standardize on fewer, more integrated platforms. A well-planned AI consolidation strategy ensures that different AI capabilities — predictive, generative, and agentic AI — work together seamlessly, rather than functioning as a disconnected patchwork of tools.
Interoperability is key. Organizations should prioritize AI platforms that integrate with their existing data infrastructure, allowing them to connect workflows across departments rather than creating siloed solutions. A phased migration strategy helps ease the transition, ensuring minimal disruption to ongoing operations while shifting from fragmented AI adoption to a more unified approach. Beyond technology, organizations must also define clear ownership for AI initiatives. Assigning responsibility to a dedicated AI function — whether within IT, operations, or a cross-functional team — ensures that AI adoption is not just an isolated project but a scalable, enterprise-wide initiative.
How to manage AI fragmentation: Establish a Center of Excellence (CoE)
A Center of Excellence (CoE) serves as a centralized hub of expertise, resources, and best practices for scaling AI initiatives. By standardizing AI implementation across the organization, a CoE helps streamline initiatives, eliminate redundancies, and prevent fragmentation — ensuring that AI projects are prioritized based on business impact and return on investment (ROI).
A successful AI CoE begins with a clear objective by defining how AI will support automation, decision-making, and operational efficiency. Instead of being confined to IT limitations, the CoE should be cross-functional, accelerating AI adoption and providing clear governance and oversight to ensure AI initiatives remain aligned with organizational goals.
Governance is critical. Organizations should establish guidelines for AI model deployment, ensuring data privacy, security, and ethical considerations are embedded in every AI initiative. A governance framework prevents biased decision-making, ensures compliance with evolving regulations, and builds trust in AI-driven processes. AI success isn’t just about implementation, it’s also about education. Organizations should promote AI literacy across teams, ensuring that employees understand how to leverage AI tools effectively.
Finally, AI initiatives should be measurable and adaptable. One way to do this is through performance tracking mechanisms such as monitoring efficiency gains or AI-driven revenue impact. Organizations that refine their AI strategies maximize the value derived from AI investments.
A strategic driver of long-term innovation
AI fragmentation poses a significant challenge, but it doesn’t have to. With a unified approach, companies can streamline AI adoption, enhance operational efficiency, and extract actionable insights from their automation efforts. By consolidating AI tools and frameworks and establishing a Center of Excellence, businesses can ensure that AI is not just another technology investment, but a strategic driver of long-term innovation.
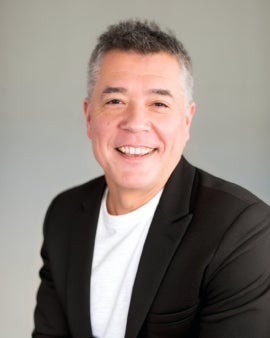
Burley Kawasaki is global VP of product marketing and strategy of Creatio, a global vendor of an AI-native platform to automate workflows and CRM with no-code.
https://assets.techrepublic.com/uploads/2025/01/tr_20250102-how-to-implement-employee-onboarding-automation.jpg
Source link
Guest Contributor